What AI can do: enumerated
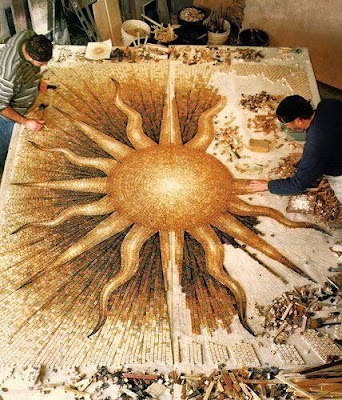
"Frankenstein was written during the first Industrial Revolution, a period of enormous changes that provoked confusion and anxiety for many. It asked searching questions about man's relationship with technology: are we creating a monster we cannot control, are we losing our humanity, our compassion, our ability to feel empathy and emotions?" This was written by Paolo Gallo, the Chief Human Resources officer at World Economic Forum. "Frankenstein relies on the notion that humans will inherently reject artificial intelligence as unnatural and bizarre. A great deal of that is owed to the particularly odd appearance of Frankenstein's monster... But what about when AI comes in a more attractive package, one that has real utility?" Now here is the ever-expanding list: - playing checkers - natural language processing - be an intelligent personal assistant - GANs (optimizing both a generator & discriminator) - DALL-E (optimizing both an embedding prior mode